Projects Last Projects
Deep Learning Approach for Tissue Segmentation in Whole Slide Images
Haneen Naaran, Amir Bishara
Supervised by Tal Neoran & Roy Velich
We investigate the use of deep learning models for the semantic segmentation of Hematoxylin and Eosin Whole Slide images (H&E WSI). H&E images are commonly used in pathology for the diagnosis.
Automated segmentation can aid in the efficient identification of different structures within these wsi images. This segmentation problem can be solved by classic computer vision algorithms, like Otsu’s thresholding method. However, this method does not generalize well on different datasets and is not robust to artifacts on scans. Hence, we studied the use of deep learning models for this segmentation task, aiming to get better generalization ability and robustness to artifacts. We trained and tested several deep learning models, including U-Net and FusionNet, in a supervised manner on datasets of H&E images that include foreground tissue segmentation performed by the OTSU’s thresholding method. We evaluated their performance using various metrics, including visual examination.
Our results show that these models can achieve high accuracy in the semantic segmentation and they generalize well on different datasets.
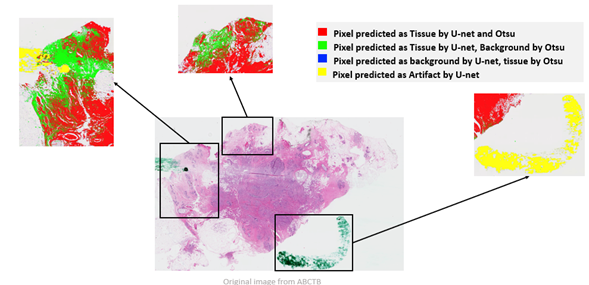
Please, see project report.
Please, see final presentation.