Projects Last Projects
Weakly Supervised Learning Methods for Analysis of Histological Whole Slide Images
Tal Neoran
Supervised by Gil Shamai
In the field of histopathology, slices of tissue samples are typically stained and examined under a microscope for diagnosis of cancer and other diseases, and for analysis of different properties of the diseases. In recent years, the use whole slide imaging technology for digital scanning of glass slides has rapidly increased, allowing the employment of artificial intelligence (AI) methods to improve the analysis and prediction abilities in computational histopathology. Scanning of a glass slide results in a scan known as a whole slide image (WSI), a gigapixel image of a tissue sample constructed from multiple different magnification resolutions. Performing analysis of WSIs using deep learning algorithms poses multiple challenges, for example due to the large size of the images, and due to the lack of availability of local annotations.
In this project, we implemented and assessed the potential of methods in weakly supervised and self-supervised deep learning to perform analysis and predict clinical outcomes from hematoxylin and eosin (H&E) stained WSIs of patients with breast cancer. We proposed and evaluated ways to deal with the challenges that arise when working with WSIs and applied our methods to a WSI dataset for classification of hormone receptor status information.
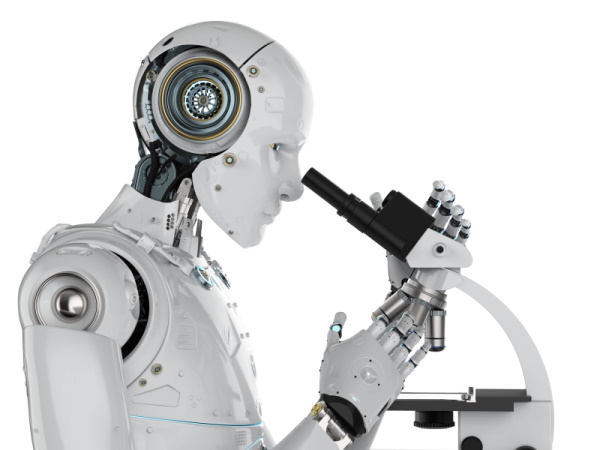
Please, see project report.
Please, see final presentation.