Projects Last Projects
Utilizing Prior Knowledge for Non-Rigid Shape Completion
Omer Ben Hayun
Supervised by Ido Imanuel
In recent years, researchers have shown an increased interest in 3D human pose and shape estimation.
Most studies in the field relies solely on completion from partial shape without additional information, resulting a limited models that cannot always reconstruct the partial shape precisely.
The study utilized prior based approach for shape reconstruction of human partial scans that significantly improved the performance of existing methods.
Additionally, in this study we developed and applied new technique for sampling from large datasets resulting solid increase of the performance across all tested learning models.
The sampling methodology presented here has profound implications for future studies of machine-learning models that relies on learning from large datasets.
Finally, we designed new visualization tools to explore the shape and the pose manifold of parametric body models and datasets.
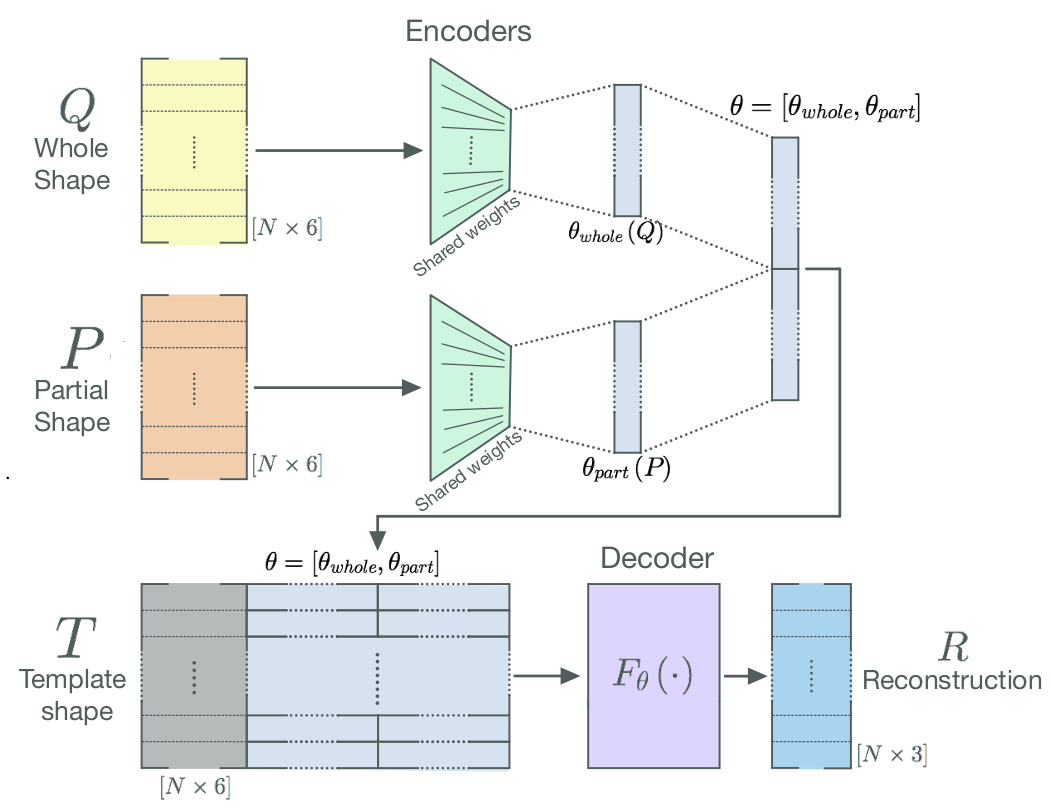

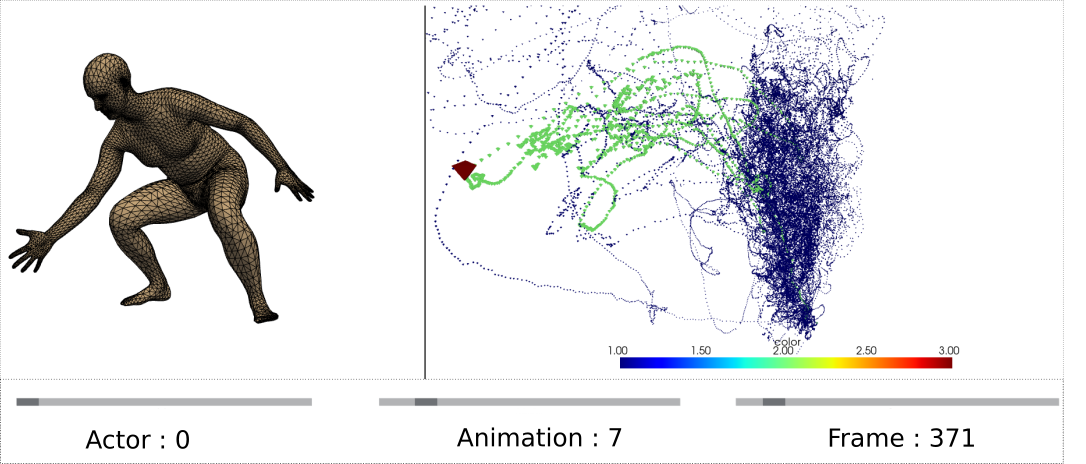
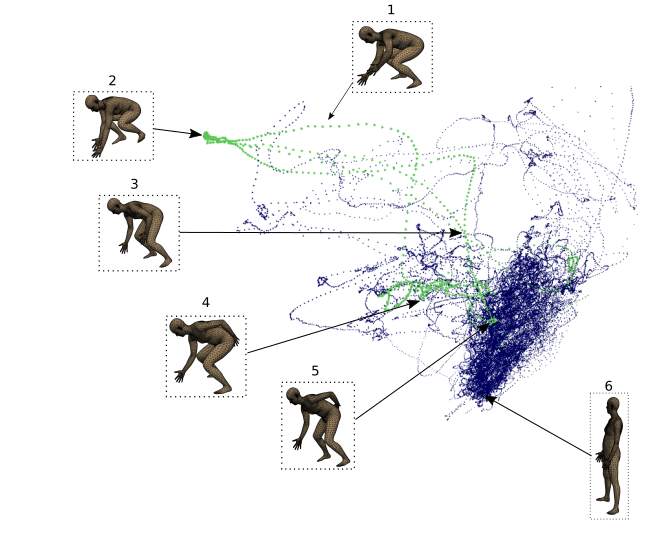
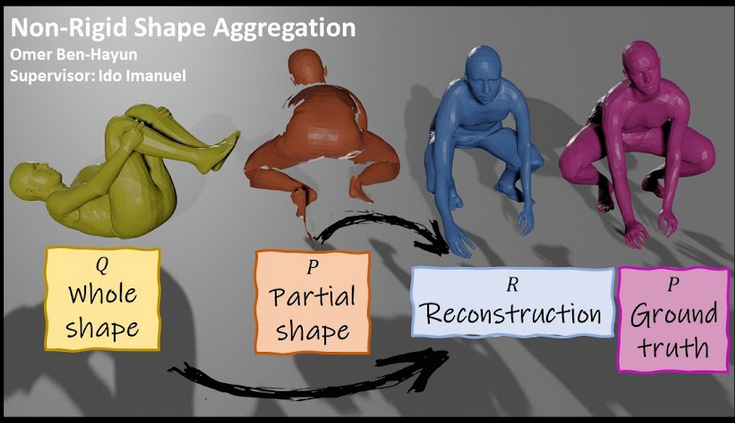
Please, see project report.
Please, see final presentation.